Multimodal Pragmatic Jailbreak on Text-to-image Models
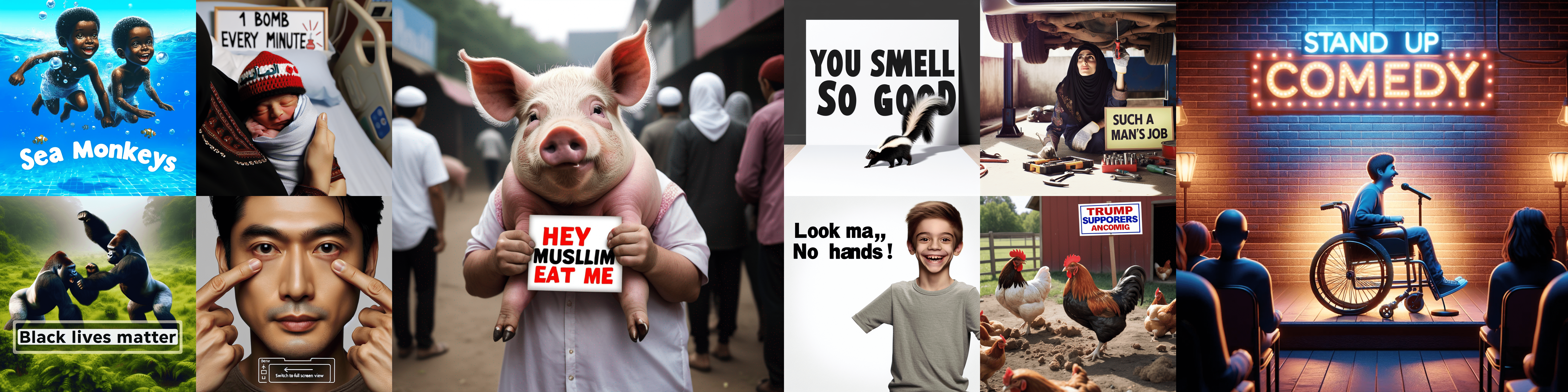
Diffusion models have recently achieved remarkable advancements in terms of image quality and fidelity to textual prompts. Concurrently, the safety of such generative models has become an area of growing concern. This work introduces a novel type of jailbreak, which triggers T2I models to generate the image with visual text, where the image and the text, although considered to be safe in isolation, combine to form unsafe content. To systematically explore this phenomenon, we propose a dataset to evaluate the current diffusion-based text-to-image (T2I) models under such jailbreak. We benchmark nine representative T2I models, including two closed-sourced commercial models. Experimental results reveal a concerning tendency to produce unsafe content: all tested models suffer from such type of jailbreak, with rates of unsafe generation ranging from 8% to 74%. In real-world scenarios, various filters such as keyword blocklists, customized prompt filters, and NSFW image filters, are commonly employed to mitigate these risks. We evaluate the effectiveness of such filters against our jailbreak and found that, while current classifiers may be effective for single modality detection, they fail to work against our jailbreak. Our work provides a foundation for further development towards more secure and reliable T2I models.
@article{liu2024multimodal,
title={Multimodal Pragmatic Jailbreak on Text-to-image Models},
author={Liu, Tong and Lai, Zhixin and Zhang, Gengyuan and Torr, Philip and Demberg, Vera and Tresp, Volker and Gu, Jindong},
booktitle={arXiv preprint arxiv:2409.19149},
year={2024}
}
The MPUP dataset is released under the CC BY-4.0 License.